The Model
unsupervised learning
This section of the study report focuses on the unsupervised learning analysis conducted using centroid (standard) and average (range) clustering methods. The Centroid clustering method groups members with more similarities within a cluster than with members of other clusters, resulting in distinct clusters that help identify their characteristics. The Average clustering method determines the best pairs of clusters based on proximity, leading to more accurate analysis.
The Centroid (Standard) clustering resulted in two clusters, with one being larger than the other. Cluster 1 has a higher number of listings per host, higher prices, and primarily consists of entire houses and apartments with more bedrooms and beds. This suggests that cluster 1 is more commercially oriented, where hosts acquire multiple properties and let them out for higher profits. This contradicts Airbnb's concept of occasional letting and sharing economy. Surprisingly, the minimum number of nights for stays in cluster 1 is smaller compared to cluster 2.
Using the Average (Range) clustering method, five clusters were formed. Cluster 4 is the largest and has a high number of listings per host (>50). Clusters 2 and 4 also exhibit more commercial characteristics, including high listing counts per host, high occupancy, more bedrooms, high availability, high prices, and consisting entirely of houses and apartments. However, the average number of years of experience for hosts in these clusters is relatively low (<4.5) compared to the other three clusters. This indicates that most hosts in clusters 2 and 4 have recently joined Airbnb with a business intention.
Overall, these unsupervised learning analyses provide insights into the clustering patterns and help identify clusters that exhibit commercialization tendencies on Airbnb.
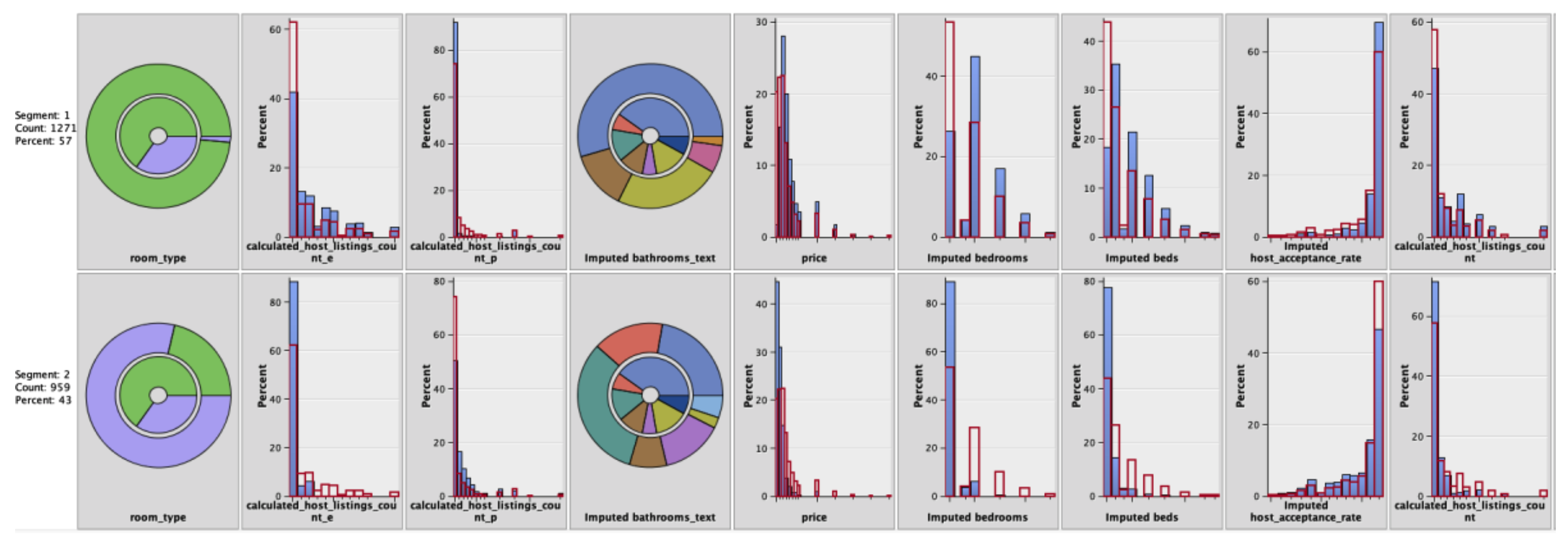
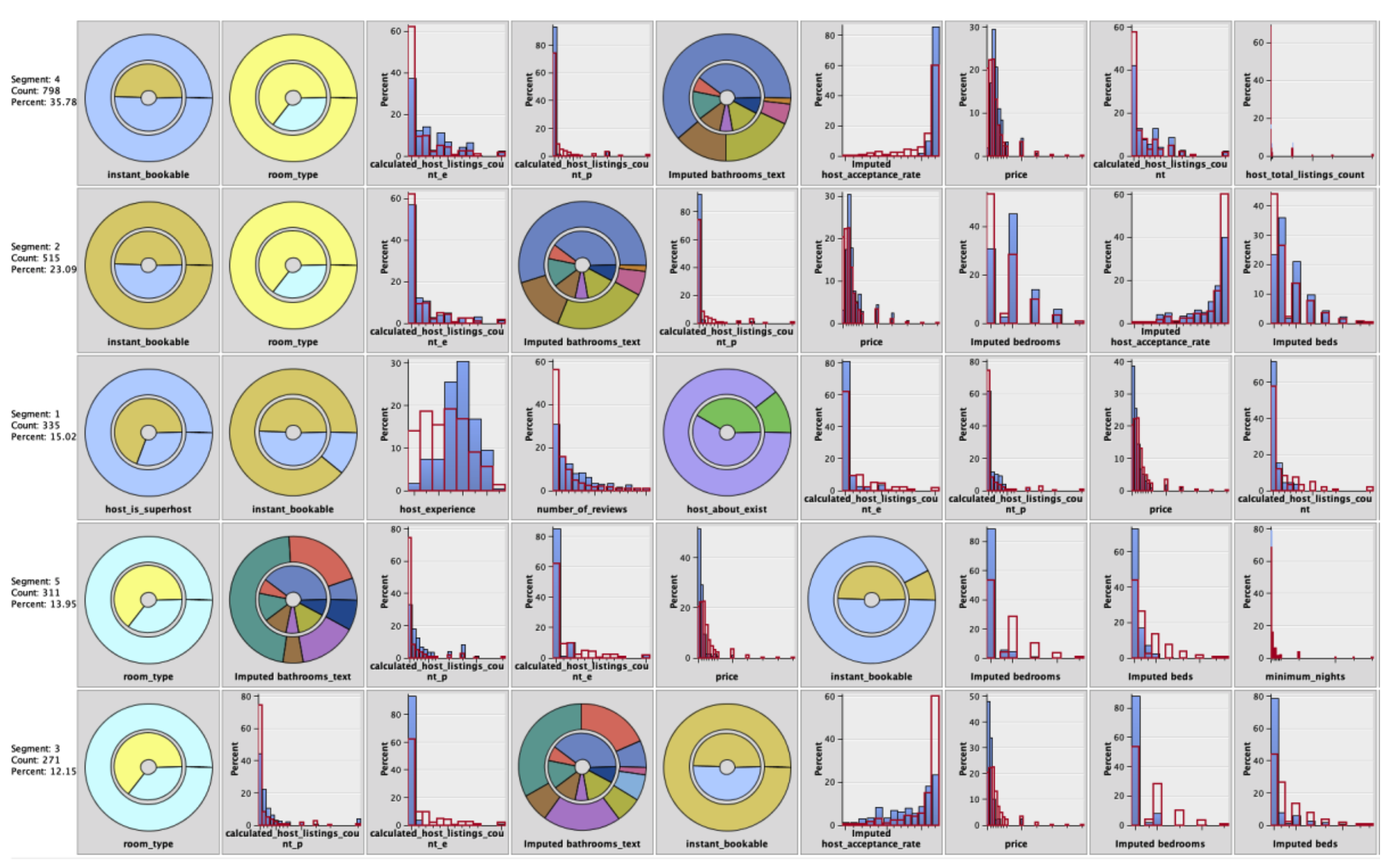